Advanced Analytics & Data
Advanced Analytics & Data
Usage of Machine Learning to transform the enterprise data assets into business value, implementing game-changing machine learning products or leveraging AI to access greater operational efficiencies.
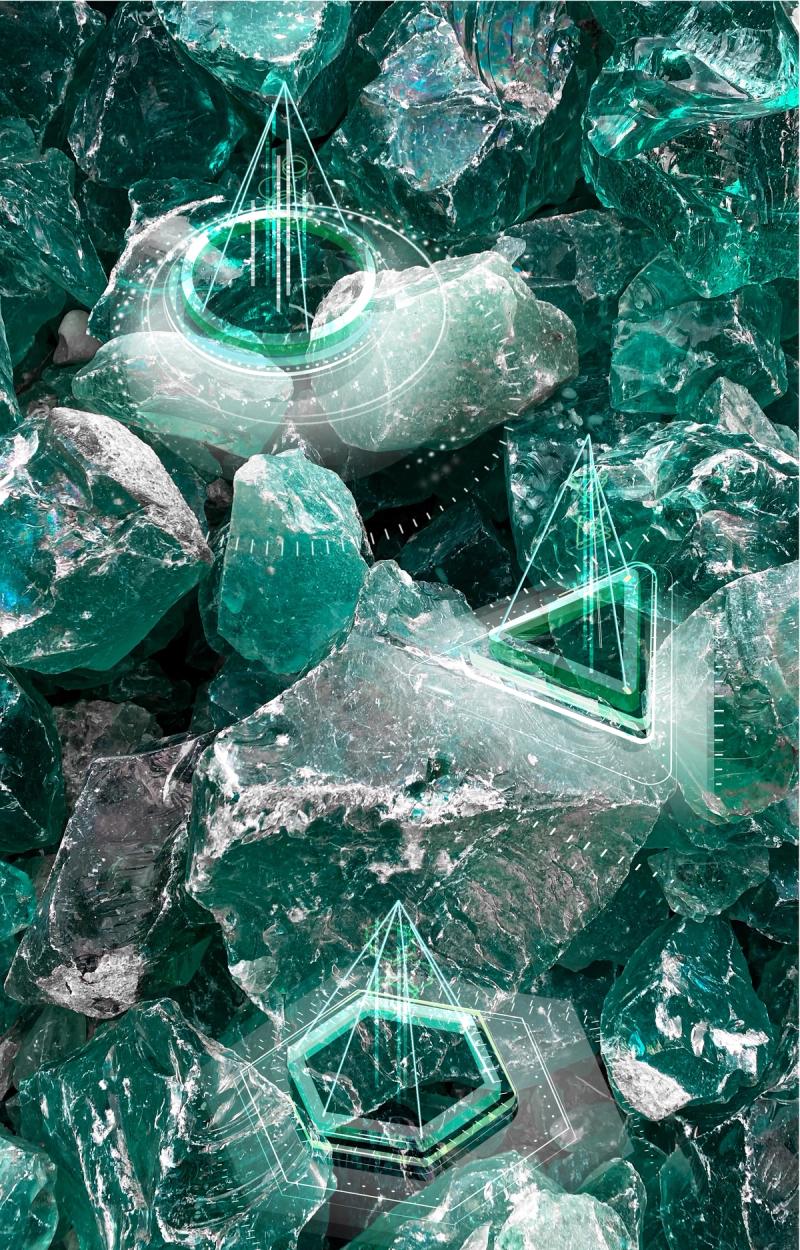
Industry Data Insight Platforms
The Platform accelerates the adoption of data-driven intelligence and AI.
- Provides framework and set of tools to kickstart AI and Data Analytics development, enables end-to-end Machine Learning and experimentation workflow;
- Allows collaboration with third-party developers with extended data protection and masking;
- Let customers and employees get access to insight through exchange;
- Runs on scalable Multi-cloud or Hybrid environment with allocation of computing resources and portability for a wide range of digital infrastructure options.
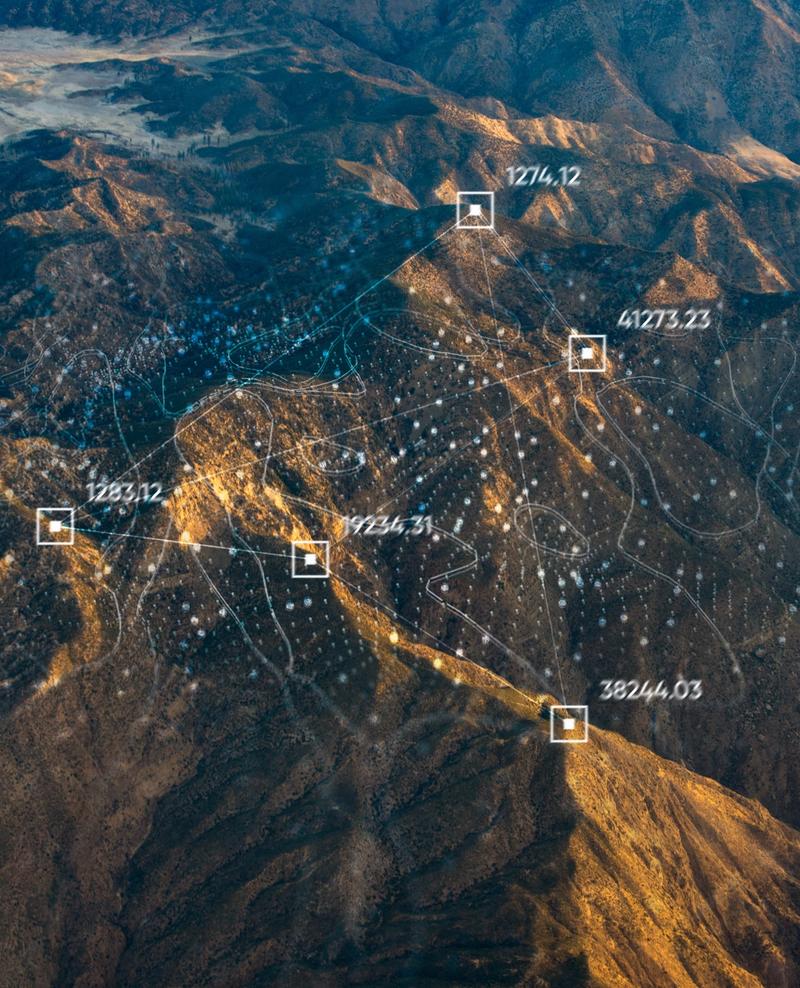
Advanced Data Analysis & Modelling
Combine heterogeneous models covering both structured (data samples, relational data, multidimensional time series) and unstructured data (text, audio, images) to provide comprehensive knowledge extraction. Calculate meta-features of target data to predict models’ accuracy and adequacy before the actual model run, advise on optimal algorithms parameters on basis of meta-knowledge base.
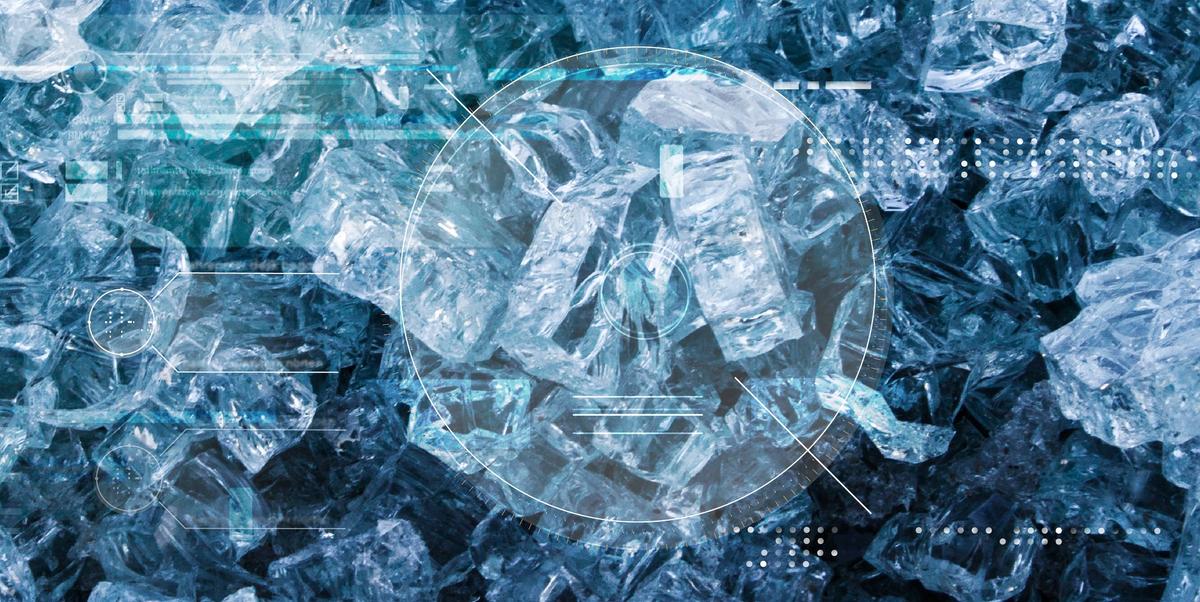
Analytical Model Validation and Audit
Model Validation and Audit is an important and sometimes mandatory step (e.g. for Finance and Insurance) to identify and address the risk of a significant deviation between the results of the model and the real-life process model represents and can lead to significant financial, and non-financial losses or loss of confidence in provided by analytical models results.
It becomes a very important step for the successful adoption of AI/ML models because AI/ML models typically act on large datasets including unstructured data (natural language, images, video). Therefore their algorithms may have a vastly larger number of parameters, which makes them more error-prone. A proper definition of the applicability domain is a tough task for such complicated models, but it becomes even more important in the case of the model designed to retrain the new coming data.
It is also vital to validate and permanently monitor model stability in real-life production use with no respect to the model nature/type/structure.
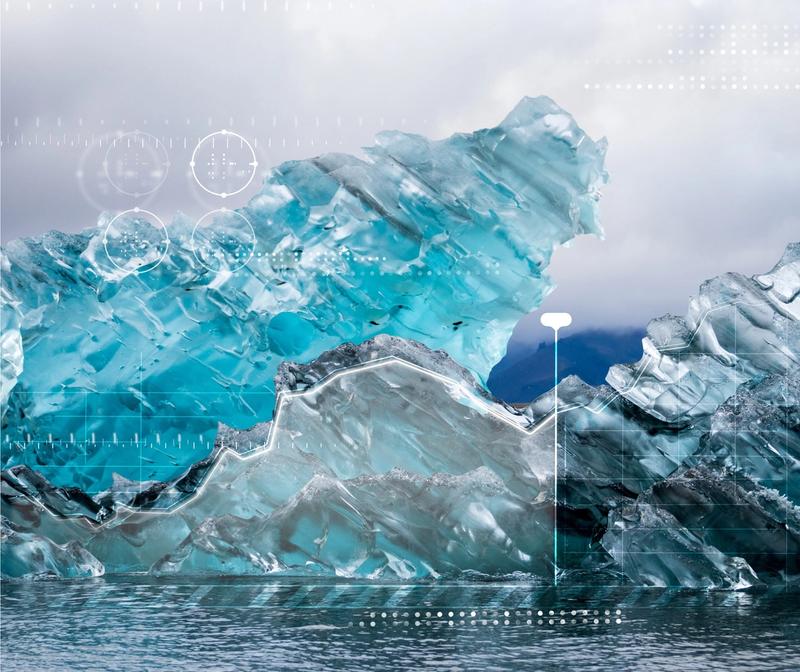
We provide Model Validation and Audit Services for third-party and internal analytics team. This is multi-discipline practice, it has methodology, technical framework and expert team to validate from purely statistical to machine learning (AI) and hybrid models for multiple industries ranging from Finance, Logistics, Mining to Life Science and Healthcare.
Wide industries coverage is possible because of the team of experts with diversified background and experience:
- Fundamental physics and mathematics;
- Mechanical- and electrical-engineering;
- Computer sciences;
- Quantitative analysis and finance.
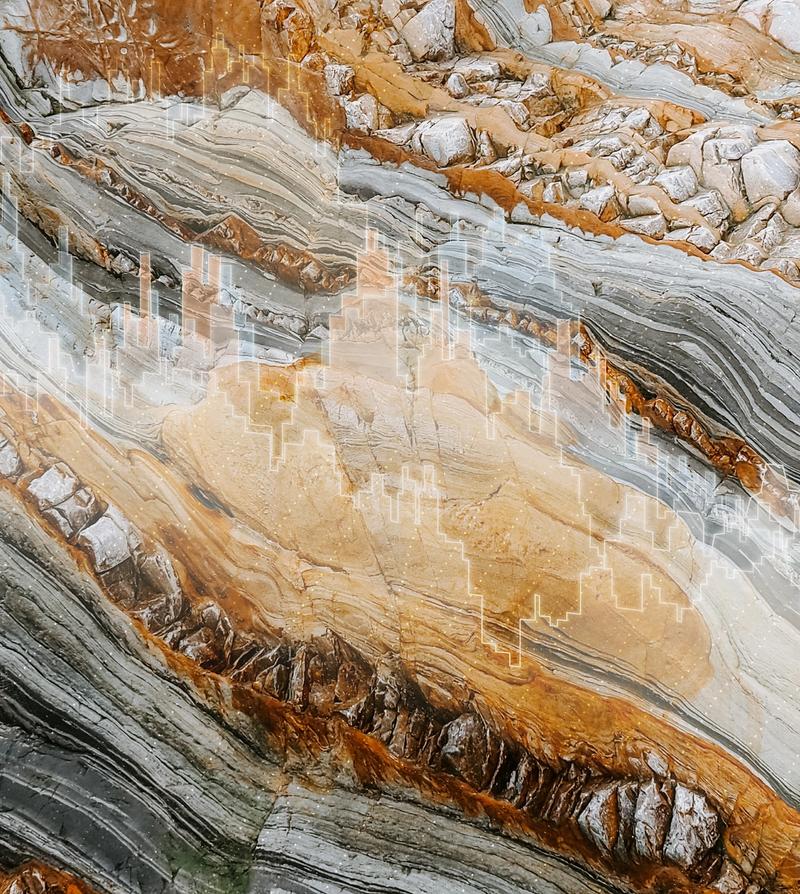
Finance and Industrial Model Development
We develop deductive stochastic models to calculate the prices of complex financial instruments. We model complex industrial and real-world processes and systems to provide optimal control and maximize revenue.
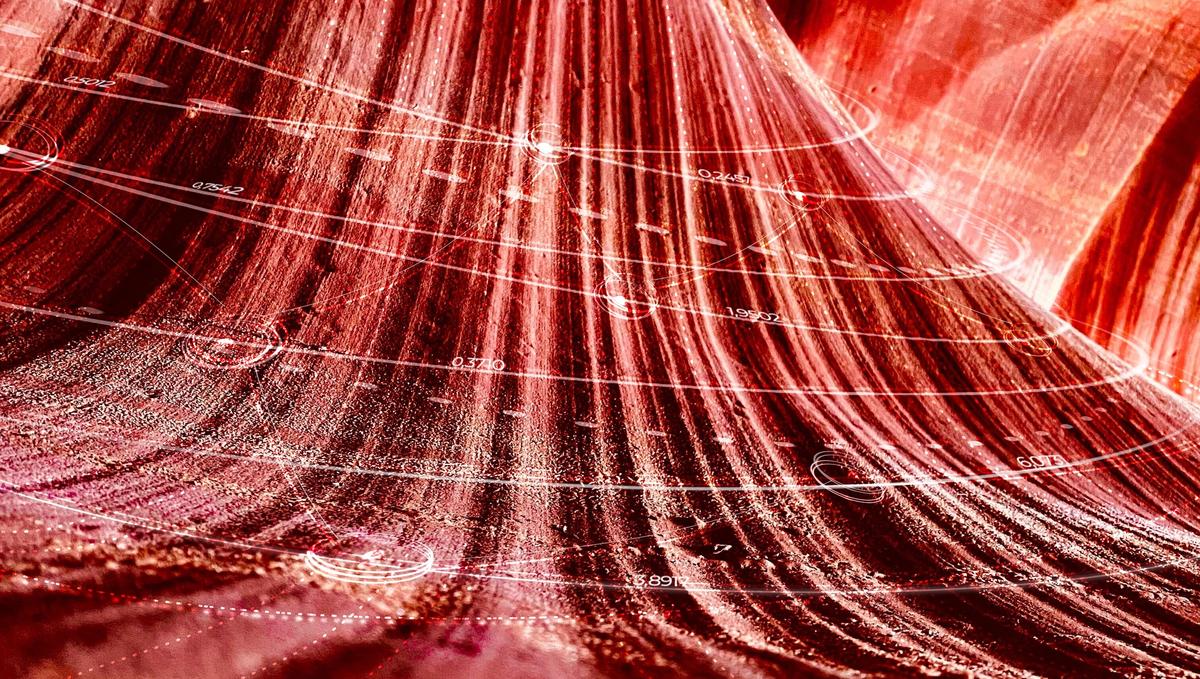
Data-driven Business
Bespoke data investigation to detect and research potentially valuable knowledge sources. Data auditing (quality, presence, security, policies). Data strategy planning (what you have and what you have missed).
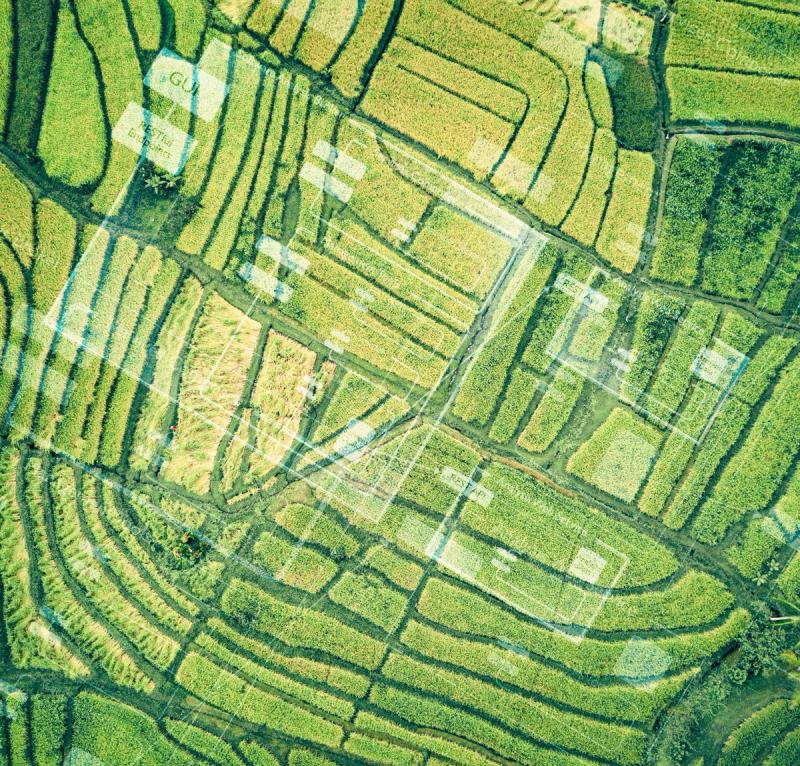
Data Management
Ontology development to bring semantics and reasoning into raw data. On-fly data enrichment based on formal reasoning on top of detailed domain ontologies.